Almost two months ago, I wrote a post on what we thought would be
thorny issues that would get in the way of importing legacy accession data into ArchivesSpace. This was mostly conjecture, because at that point, we hadn't actively been trying to move data from Beal (a homegrown FileMaker Pro database) to ArchivesSpace. Now that we've had some time to play around with importing legacy accession data, we thought you might like an update on our plan.
The Big Squeeze
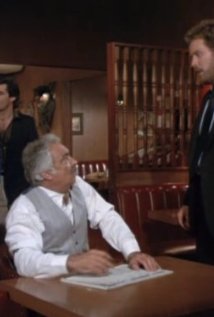 |
Unlike the actual episode from Season 3 of the A-Team, our big squeeze does not involve loan sharks, mob extortion bosses, breaking fingers, sniper assaults (phew!), or Irish pubs (or does it...). |
First things first (and this is important), we came up with a name for this project: "The Big Squeeze." Not only is this a nod to an
actual episode from Season 3 of the A-Team (the name we've given to the crew here working on ArchivesSpace implementation, that is, the ArchivesSpace Team, or simply, the A-Team), but, as you'll see, it actually fits for some of the way we'll approach importing legacy accession data into ArchivesSpace, namely, squeezing a number of disparate Beal fields into single ArchivesSpace fields.
Big Decision Early On: Don't Worry, Be Happy
Early on, we made the important decision that we simply weren't going to worry about accession records as much as we worried about EADs--we certainly weren't going to spend as much time cleaning them as we did, and still sometimes do, with our EADs. Here's our reasoning: Accession records are relatively static, and serve as mostly internal evidence of a particular transaction or event. EADs, on the other hand, are ever-changing and evolving portals to our collections for humans (internal ones, like archivists, and external ones, like researchers) and systems (and, now, other integrated systems, like DSpace and Hydra!). Likewise, going forward we simply won't be using individual accession records as frequently or even in the same way as we will individual archival descriptions (there's one exception to this which I'll talk about later).
As a result, there may be differences, even significant ones, between our legacy conventions and conventions going forward in ArchivesSpace for recording accession information. Our legacy accession records may not be super machine-readable. They won't be connected to associated finding aids. They'll be a little dirty (the horror!). We'll be using some user-defined fields that we won't use going forward. Rather than making significant changes to ArchivesSpace or the accessions importer, we'll just be squeezing a number of Beal fields into one ArchivesSpace field. We'll use Boolean fields rather than create events for some information. We'll use free text fields rather than create actual locations (at least for now). And we're OK with all this. Dare I say we're actually happy with this decision...
The Plan, Stan
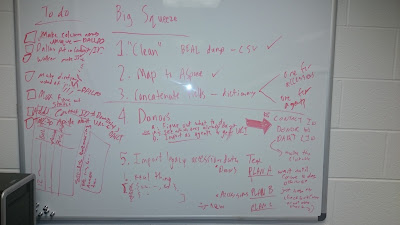 |
The plan. Ignore that stuff on the bottom left. |
As you may or may not be able to read in the image above (I don't have the best handwriting), we've formulated a six-step plan:
- "Clean" and prep a data dump from Beal.
- Map Beal fields to ArchivesSpace fields
- Build the JSON that ArchivesSpace expects.
- Deal with donors.
- Do a dry run.
- Do the real thing.
Simple, right?
"Clean" and Prep a Data Dump from Beal (Our Homegrown FileMaker Pro Database)
First things first, we need to get our accession data out of our current database. This is a fairly straightforward process, since exporting records from FileMaker Pro is as simple as File --> Export Records, choosing a file name and type, and specifying fields and order for export. I say "fairly" straightforward because of some little quirks about FileMaker Pro we've learned along the way, such as the fact that CSV exports don't include header information, but that there's a mysterious file extension ".mer" that does, that exports contain a bunch of null characters which lead to encoding errors, and that the CSV/MER export by default has a very strange way of recording multiple values in the same field, namely, that it throws the second, third, fourth, etc., values onto the next line(s), all by themselves (and since the CSV reader object in Python is not iterable, it gets tricky to associate orphan values with their proper record).
Then, there's "cleaning" and prepping. Cleaning is in quotes because we aren't actually cleaning all that much. Mostly, "cleaning" is more like straightening, not spring or deep cleaning, preparing the accession records by distilling them down to only the essentials. We made header column names unique (because there were some that weren't), we removed blanks rows, we identified and weeded out meaningless (read, information-less) records, and we filled in missing date information (ArchivesSpace accession records require dates) in most instances by writing a program that automatically guesses a date using context clues and, for about 30 or so, filled them in by hand. In some instances, we also normalized some values for fields with controlled vocabularies--nothing major, though.
Since we're still actively accessioning materials, we're trying to do this in way that will allow us to easily replicate it on future exports from Beal.
You can read through the code Dallas used to do most of this
here; date guessing is
here.
Map Beal Fields to ArchivesSpace Fields
Again, this process was fairly straightforward, especially once we made the decision not to worry too much about differences in the ways that Beal and ArchivesSpace model and expect all the components that make up an accession record.
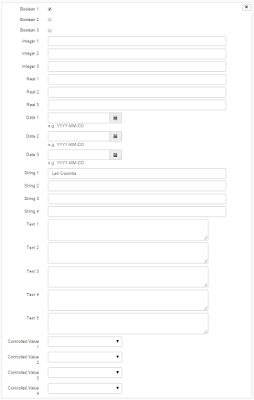 |
We'll be making fairly extensive use of the user-defined portion of ArchivesSpace accession records. Has anyone changed the labels for these fields? If so, let us know! We're keen to explore this. |
We broke this down into a number of categories:
- Basic Information, like a date and description for an accession, it's size, provenance information, and basic events associated with it, such as whether or not it's receipt has been acknowledged (here's an example of a case where we'll use a Boolean rather than create an event, even though going forward we'll probably use an actual ArchivesSpace event).
- Separations, or information about parts of collections we decided not to keep. Here we're talking about fields like description, destruction or return date, location information and volume. When we do have a date associated with a separation, we'll create an actual deaccession record. If we don't, we'll just squeeze all this information into the basic disposition field in ArchivesSpace (again, probably not how we'll use that field going forward, but that's OK!).
- Gift Agreement, the status of it, at least. We'll be using a user defined controlled vocabulary field.
- Copyright, like information on whether it has been transferred. We're going with the tried and true Conditions Governing Use note, although we've been doing a lot of talking lately about how we'll record this type of information going forward, especially for digital collections, and how the rights module in ArchivesSpace, which we'd like to be the system of record for this type of information, isn't really set up to take in full PREMIS rights statements from Archivematica (or RightsStatements.org rights, for that matter) [1].
- Restrictions, which will go to a Conditions Governing Access note (although that same discussion about rights from above applies here as well).
- Processing, that is, information we record upon accession that helps us plan and manage our processing, information like the difficulty level, the percentage we expect to retain, the priority level, the person who will do it, any notes and the status. Mostly we'll take advantage of the Collection Management fields in ArchivesSpace, and for that last one (the exception I mentioned earlier), we're hopeful that, after an ongoing project to actually determine the status for many backlogged collections, we'll be able to use the events "Processing in Progress" and "Processing Completed". Our thought process here is that this is an instance where we actually will be using accession records actively going forward to determine which accessions still need to be processed; we didn't want different conventions for this pre- and post-ArchivesSpace migration. If that doesn't work out, or it turns out that it will take too much time to straighten out the backlog, we'll be using another user-defined controlled vocabulary field.
- Unprocessed Location, which will, in the interim, just go to a user-defined free text field. This information has not been recorded consistently, and in the past we haven't been as granular with location information as ArchivesSpace is. We actually like the granularity of location information in ArchivesSpace, though, especially with the affordances of the Yale Container Mangement Plug-in and other recent news. So this is definitely something that will be different going forward, and may actually involve extensive retrospective work.
- Donors, their names and contact information, as well as some identifiers and numbers we use for various related systems. More on that later. This is also an exception, an instance where we did decide to make a significant change to ArchivesSpace. Check out this post to learn more about the Donor Details Plug-in.
- Leftovers, or information we won't keep going forward. Hopefully we won't regret this later!
One thing we're considering is trying to make it obvious in ArchivesSpace which records are legacy records and which aren't, perhaps making them read only, so that if conventions change for recording a particular type of information because of the new ArchivesSpace environment, it will be less likely (but not impossible) that archivists will see the old conventions and use those as a model.
You can check out our final mapping
here.
Build the JSON that ArchivesSpace Expects
If you've never played around with it, JSON is awesome. It's nothing fancy, just really well-organized data. It's easy to interact with and it's what you get and post from ArchivesSpace and a number of other applications via their API.
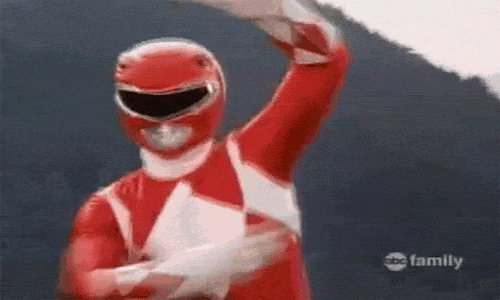 |
Another famous JSON Jason! [2] |
In that mapping spreadsheet you can see how we've mapped Beal fields to the JSON that ArchivesSpace expects. Essentially this involves reading from the CSV and concatenating certain fields (in a way that we could easily parse later if need be), that is, the big squeeze, then making ArchivesSpace deaccession, extent, collection management, user-defined, external documents, date, access restrictions, etc., lists and then JSON.
You can take a look at that code from Walker
here.
Deal with Donors
Donors get their own section. In the end, we hope to do donor agents for accessions the
same way we did subject and creator agents (as well as other types of subjects) for EADs, importing them first and linking them up to the appropriate resource/accession later. This won't be too hard, but there are some extenuating circumstances a number of moving parts to this process.
We still have some work to do with some associates of the A-Team here matching up donor information from Beal with donor information from a university system called Dart which is used to track all types of donations to the university, This is a little tricky because we're not always sure which system has the most up-to-date contact information, and because we're not always if Samuel Jackson and Samuel L. Jackson, for example, are the same person (but seriously, we don't have anything from Samuel L. Jackson). The Donor Details plug-in will help us keep this straight (once we're using it!), but in the interim, we have to go through and try to determine which is which.
We're also currently deciding whether we should actually keep contact information in ArchivesSpace or just point to Dart as the system of record for this information, which is on a much more secure server than ArchivesSpace will be. That presents some problems, obviously, if people need contact information but don't have access to Dart, but we've had internal auditors question our practice of keeping this type of sensitive information in Beal, for example. So that's still an open issue.
Do a Dry Run
It's been done! And it works! It only took an hour and twenty some minutes to write 20,000 accessions (albeit, without donor information)! If you go to the build the JSON
link you can also get a peak at how we used the ArchivesSpace API to write all this data to ArchivesSpace. Dallas has also written an excellent post on
how to use the ArchivesSpace API more generally which you should also check out.
Do the Real Thing
Sometime between now and April 1, 2016, at the end of our grant, when all the stars align and all the moving parts converge (and once, as a staff we're ready to go forward with accession material and describing it in ArchivesSpace), we'll import or copy legacy accession data into our production environment (this is, by the way, also after we have a production environment...). We're thinking now that we might go live with resources in ArchivesSpace before we go live with accessions, but no firm dates either way as of yet.
Conclusion
Well, that's the plan! What do you think? How did you approach accessions? Are we committing sacrilege by taking a "don't worry, be happy" approach? Let us know via
email or on
Twitter, or leave a comment below!
[1] Thanks, Ben, for bringing that to our attention.
[2] Stolen from the Internet: http://www.snarksquad.com/wp-content/uploads/2013/01/redranger.gif